减法优化器(Subtraction-Average-Based Optimizer,SABO)是2023年刚出的智能优化算法。目前知网中文期刊基本搜不到,并且可以遇见未来一年文章也很少。SABO算法原理简单,算上初始化粒子,总共不超过6个公式。但是SABO的寻优效果却非常好。SABO设计的基本灵感来自数学概念,如平均值、搜索代理位置的差异以及目标函数的两个值的差异符号。
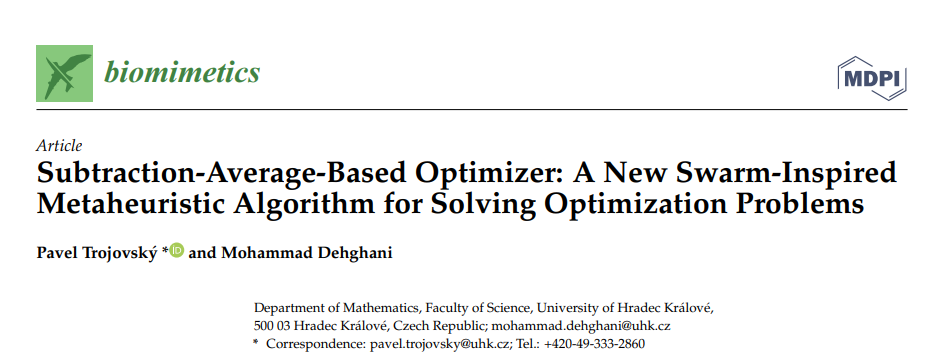
This paper presents a new evolutionary-based approach called a Subtraction-Average-Based Optimizer (SABO) for solving optimization problems. The fundamental inspiration of the proposed SABO is to use the subtraction average of searcher agents to update the position of population members in the search space. The different steps of the SABO’s implementation are described and then mathematically modeled for optimization tasks. The performance of the proposed SABO approach is tested for the optimization of fifty-two standard benchmark functions, consisting of unimodal, high-dimensional multimodal, and fixed-dimensional multimodal types, and the CEC 2017 test suite. The optimization results show that the proposed SABO approach effectively solves the optimization problems by balancing the exploration and exploitation in the search process of the problem-solving space. The results of the SABO are compared with the performance of twelve well-known metaheuristic algorithms. The analysis of the simulation results shows that the proposed SABO approach provides superior results for most of the benchmark functions. Furthermore, it provides a much more competitive and outstanding performance than its competitor algorithms. Additionally, the proposed approach is implemented for four engineering design problems to evaluate the SABO in handling optimization tasks for real-world applications. The optimization results show that the proposed SABO approach can solve for real-world applications and provides more optimal designs than its competitor algorithms.
(参考翻译)本文提出了一种新的基于进化的方法,称为基于减法平均的优化器(SABO)来解决优化问题。提出的SABO的基本灵感是使用搜索代理的减法平均来更新种群成员在搜索空间中的位置。描述了SABO实现的不同步骤,然后对优化任务进行数学建模。提出的SABO方法的性能进行了测试,以优化52个标准基准函数,包括单峰、高维多峰和固定维多峰类型,以及CEC 2017测试套件。优化结果表明,提出的SABO方法在求解空间的搜索过程中平衡了探索和利用,有效地解决了优化问题。将SABO算法的结果与12种知名的元启发式算法的性能进行了比较。仿真结果分析表明,所提出的SABO方法对大多数基准函数都有较好的求解效果。此外,它提供了比竞争对手更具竞争力和突出的性能。此外,将该方法应用于四个工程设计问题,以评估在处理实际应用中的优化任务时的SABO。优化结果表明,所提出的SABO方法可以解决实际应用问题,并提供比其竞争算法更多的优化设计。
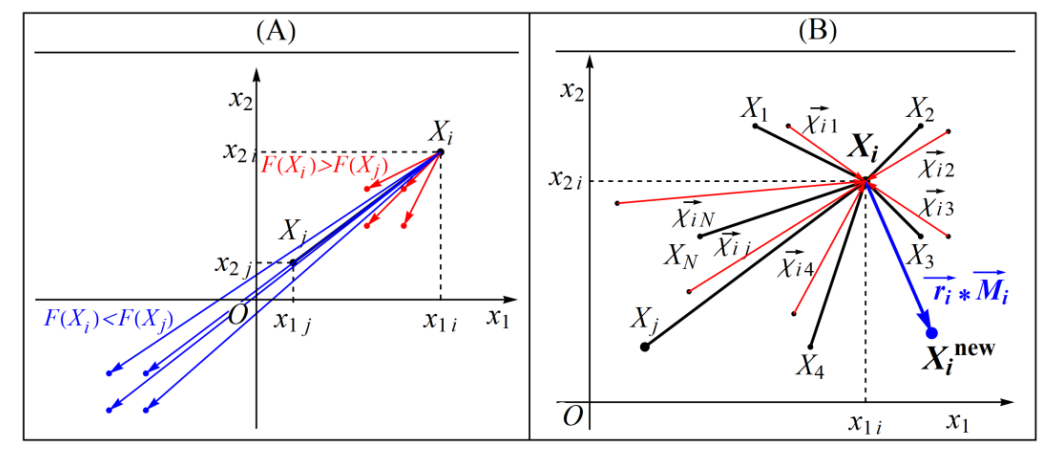
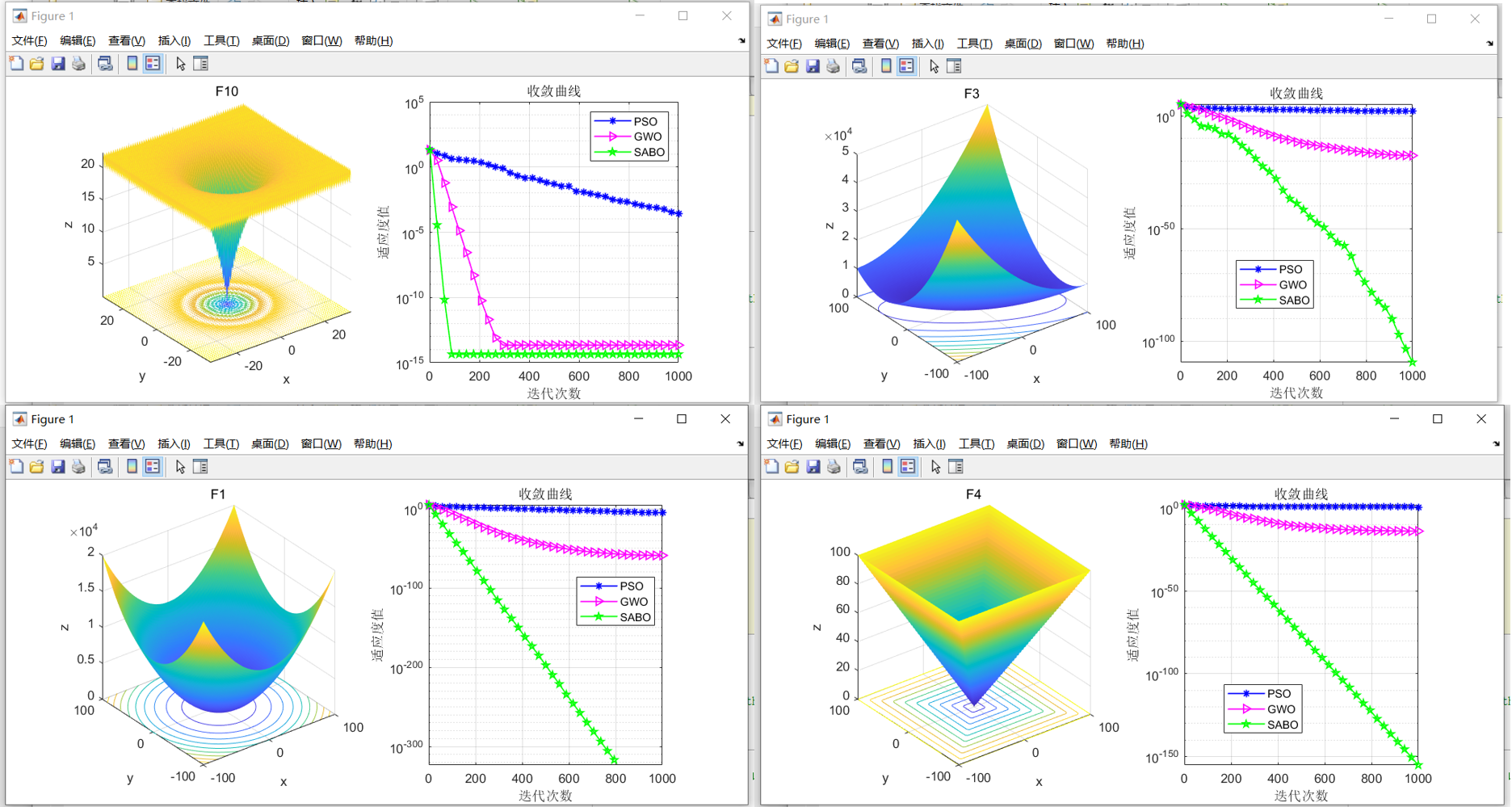